American Express - Default Prediction
대회 : https://www.kaggle.com/competitions/amex-default-prediction/overview
American Express - Default Prediction
Predict if a customer will default in the future
www.kaggle.com
노트북 : https://www.kaggle.com/code/pavelvod/27-place-sequentialencoder
[27 place] SequentialEncoder
Explore and run machine learning code with Kaggle Notebooks | Using data from American Express - Default Prediction
www.kaggle.com
배경
신용 부도 예측을 통해, 소비자에게 대출을 해주는 비즈니스에서 리스크를 관리할 수 있다.
대출하는 기관이, 대출을 해줄지 말지 결정하는 것을 최적화 할 수 있다.
이걸 통해 더 나은 고객 경험과, 건전한 비즈니스 경제성으로 이어진다.
현재 존재하는 모델은 리스크 관리를 위해 존재한다.
그러나, 이보다 더 나은 성능을 발휘할 수 있는 모델을 알 수 있다.
아메리칸 익스프레스 : 세계적인 payments 회사
해당 대회에서는, 머신러닝을 통해 신용 불이행을 예측한다.
학습 / 검증 / 테스트 데이터셋에는 Time-series behavioral 데이터와, 익명화된 고객 프로필 정보가 포함된다.
Features 를 생성하는 것부터, 좀 더 유기적인 방식으로 데이터를 이용해 모델을 사용하는 것까지,, 가장 강력할 모델을 만들기 위한 다양한 테크닉을 살펴볼 수 있다.
신용 불이행 예측 모델에 도전해보자!
평가방법
M : evaluation metric 은, rank ordering 에 쓰이는 G 와 D 에 대한 평균이다.
G : Normalized Gini Coefficient
D : default rate captured at 4%
- 예측들 중 가장 높은 랭크인, 4% predictions 에서 포착된, positive label 의 비율을 뜻함.
- Sensitivity/Recall 통계를 나타낸다.
M = 0.5 * (G + D)
그리고 G 와 D 에 대해, negative label 에는 다운 샘플링을 조정하기 위해 20의 가중치가 부여된다.
따라서, M 의 최대값은 1.0 이다.
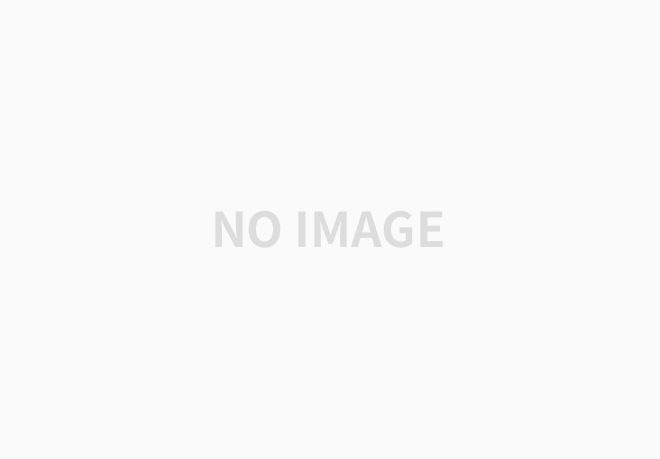
제출 파일
테스트 데이터셋의 customer_ID 에 대해, target variable 에 대한 probability 를 예측해야한다.
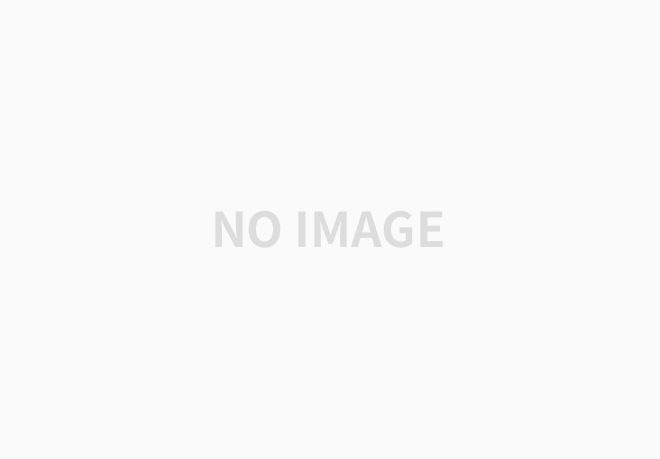
데이터셋
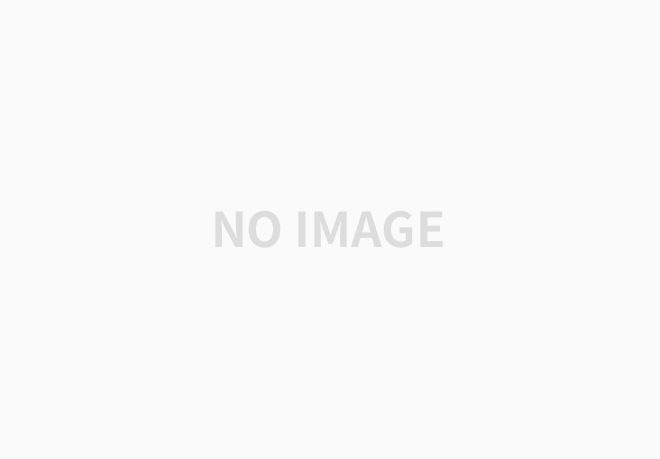
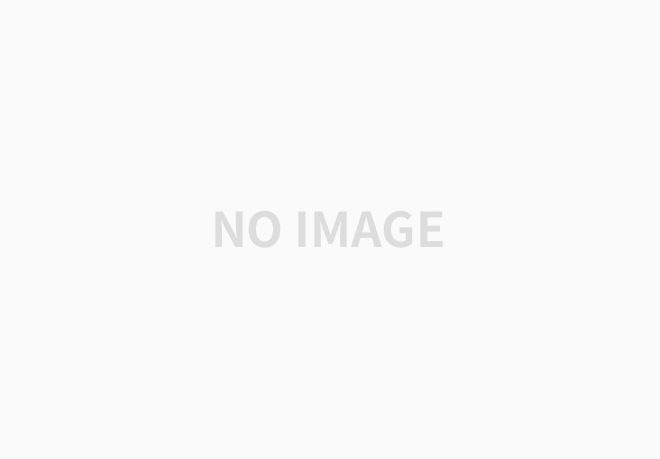
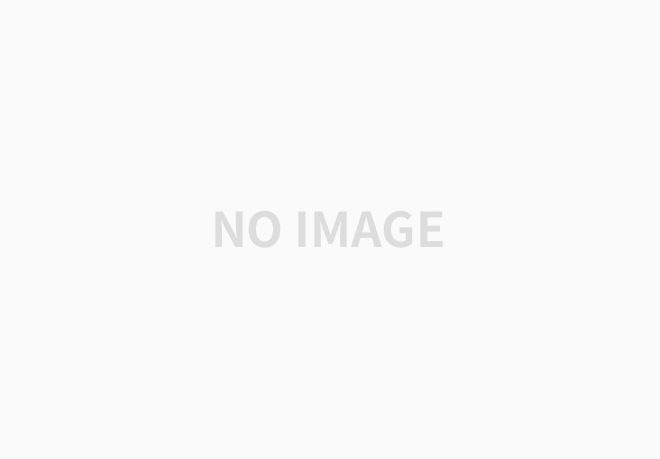
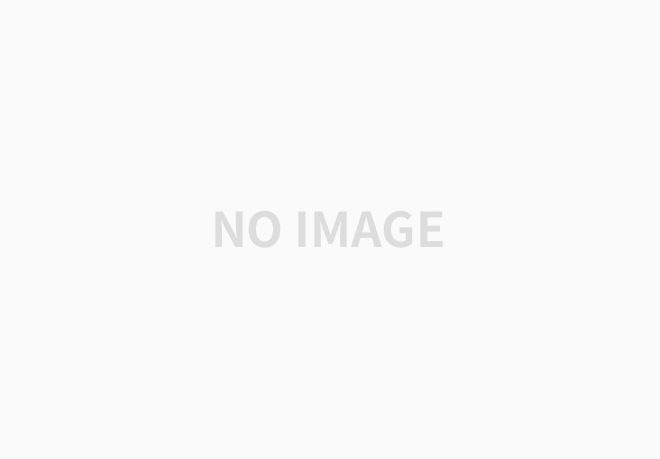
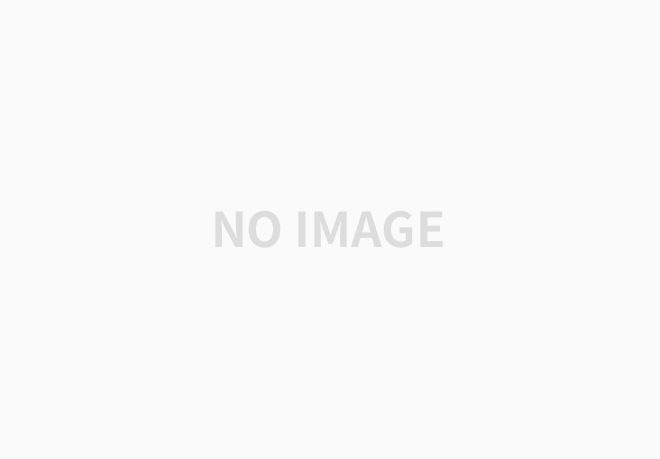
train data 를 이용하여, rank 나 month 등 새로운 featuere 생성
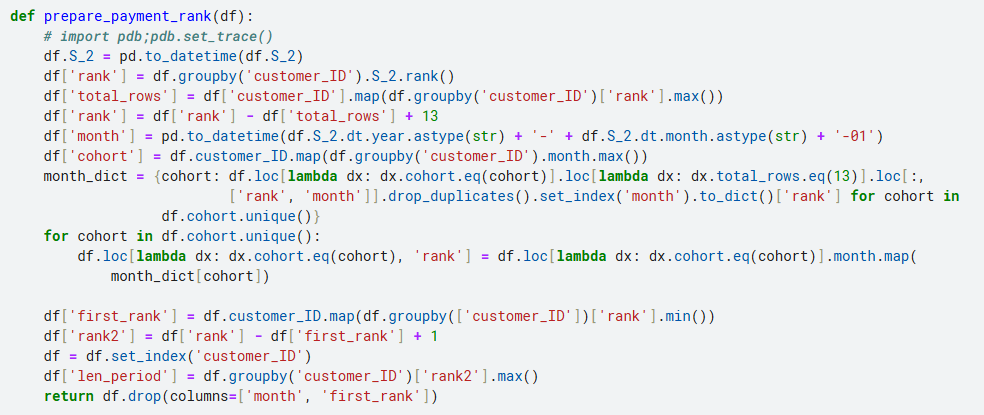
여러개의 Feature 를 이용한 새로운 feature 생성
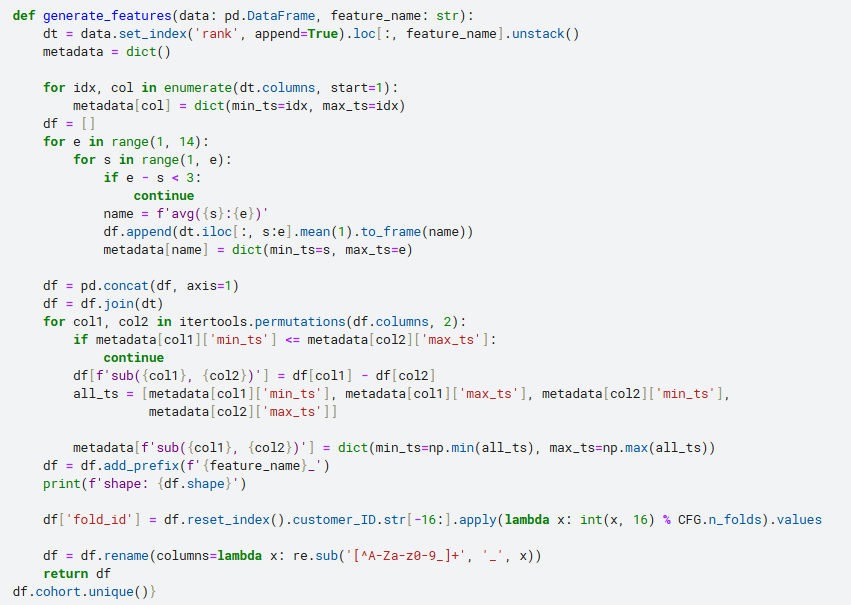
data 에 'rank' 라는 값으로 인덱스를 만들어서, feature_name 열을 기준으로 df 를 재구성하고,
unstack 으로 time-series 데이터를 행끼리 정렬함
데이터셋 하루종일 불러오는 중이라 구조는 이후에 정리할 수 있을 듯함 (GPU 로 불러오는데도 엄청 오래 걸리넹)
Sequential transformer
class SequentialTransformer: def __init__(self, seed, n_folds, target, feature_name): self.models = [] self.seed = seed self.n_folds = n_folds self.target = target self.feature_name = feature_name self.features = [] def fit(self, X, y): df = X.join(y) self.features = [col for col in df.columns if col not in ['fold_id', 'target']] params = { 'objective': 'binary', 'metric': "binary_logloss", 'seed': self.seed } print(f'numerical features ({len(self.features)}): ') for i in range(0, len(self.features), 10): print(f'\t{self.features[i:i + 10]}') oof = [] for fold_id in range(self.n_folds): print(' ') print('-'*50) print(f'>>> Training fold {fold_id} w. {len(self.features)} features :') x_trn = df.loc[lambda dx: dx.fold_id.ne(fold_id), self.features] x_val = df.loc[lambda dx: dx.fold_id.eq(fold_id), self.features] y_trn = df.loc[lambda dx: dx.fold_id.ne(fold_id), self.target] y_val = df.loc[lambda dx: dx.fold_id.eq(fold_id), self.target] lgb_train = lgb.Dataset(x_trn, y_trn) lgb_valid = lgb.Dataset(x_val, y_val) model = lgb.train(params=params, train_set=lgb_train, num_boost_round=100000, valid_sets=[lgb_train, lgb_valid], early_stopping_rounds=50, verbose_eval=100 ) val_pred = model.predict(x_val) score = amex_score(y_val, val_pred) print(f'fold {fold_id} CV score : {score}') oof.append(pd.DataFrame(val_pred, index=x_val.index, columns=[self.feature_name])) self.models.append(model) oof = pd.concat(oof) r = oof.join(y) print(f'amex_score : {amex_score(r.target, r[self.feature_name])}') return self def predict(self, X): predictions = [] for model in self.models: predictions.append(pd.DataFrame(model.predict(X.loc[:, self.features]), index=X.index, columns=[self.feature_name])) predictions = pd.concat(predictions).groupby(level=0).mean() return predictions
LightGBM 모델을 이용하여 n_fold Cross Validation 방식으로 예측을 하였다.
이진 분류 문제이기 때문에, LightGBM 의 params 를 아래와 같이 세팅하였다.
- objective : binary
- metric : binary_loss
CV 모델이기 때문에, validaion data 에 대한 oof 예측값을 구하였다.
(예전에 했던 기억으로는 CV 저렇게 id 지정해줄 필요가 없었어서... 데이터 불러와지면 다시 찾아봐야 할듯함)
amex_score 는 위의 Evaluation 에서 언급 했던 공식이다.
요약
신용 데이터를 이용해서 feature_set 을 만들고
LightGBM 모델을 사용했고,
train set 과 valid set 으로 나눠서
Cross validatoin 방식으로 OOF 예측값들을 이용하여 최종 prediction 을 만든 다음에
이를 이용하여 학습하는 방식으로 학습을 진행하였다.
Lesson-Learned
가장 중요한 부분은 feature 를 생성하는 부분인 것 같다.
train_data 를 보면 customer_id 가 여러개가 있었는데, 이를 groupby 해서 rank 등의 feature 를 만들고,
이 feature 들을 이용하여 새로운 feature 들을 만들었다.
기존 노트북에 실행 결과도 없고, 노트북에서도 아직도 데이터를 불러오는 중이라 결과는 나중에 첨부할 예정
-
이라고 했었는데 시간 다 써서 실행이 안됨... 주말에 colab 으로 다시 해야하나
참고
https://lightgbm.readthedocs.io/en/latest/Parameters.html
댓글을 사용할 수 없습니다.